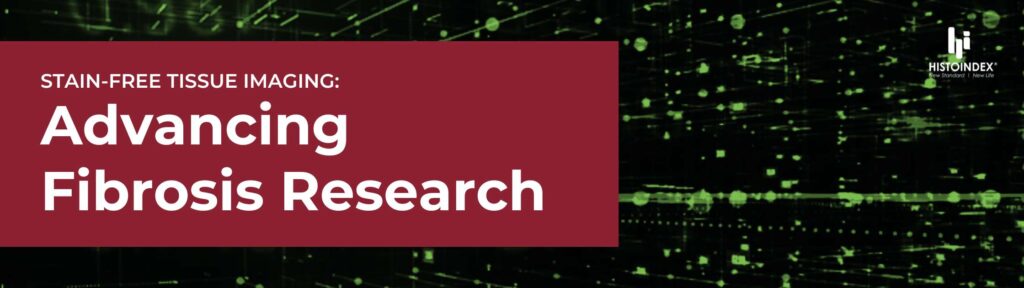
Introduction
- What stain-free imaging is, and techniques like second harmonic generation
- How it is different from traditional staining methods, and its advantages
- Its applications in fibrosis research
- Emerging trends that may shape the future of this field.

What is Stain-Free Tissue Imaging?
Stain-free tissue imaging refers to a category of imaging methods that allow for the visualization and analysis of tissue samples without using traditional chemical stains. Second Harmonic Generation (SHG) microscopy is a prominent technique in this category.
SHG is an optical process that occurs in certain materials, including collagen, the main biomarker and a key structural protein involved in fibrosis. In SHG microscopy, high-intensity light interacts with non-centrosymmetric structures in the tissue, generating light at exactly twice the incident frequency. This phenomenon allows for the visualization of collagen fibers without needing exogenous labels or stains.
Techniques like SHG microscopy offer an alternative to traditional staining methods for tissue analysis. Traditional tissue imaging relies on chemical staining to differentiate between various tissue components. Standard staining techniques used in fibrosis assessment include Hematoxylin and Eosin (H&E), Masson’s Trichrome, and Picosirius Red staining. These methods involve multiple steps, including fixation, dehydration, staining, and mounting.
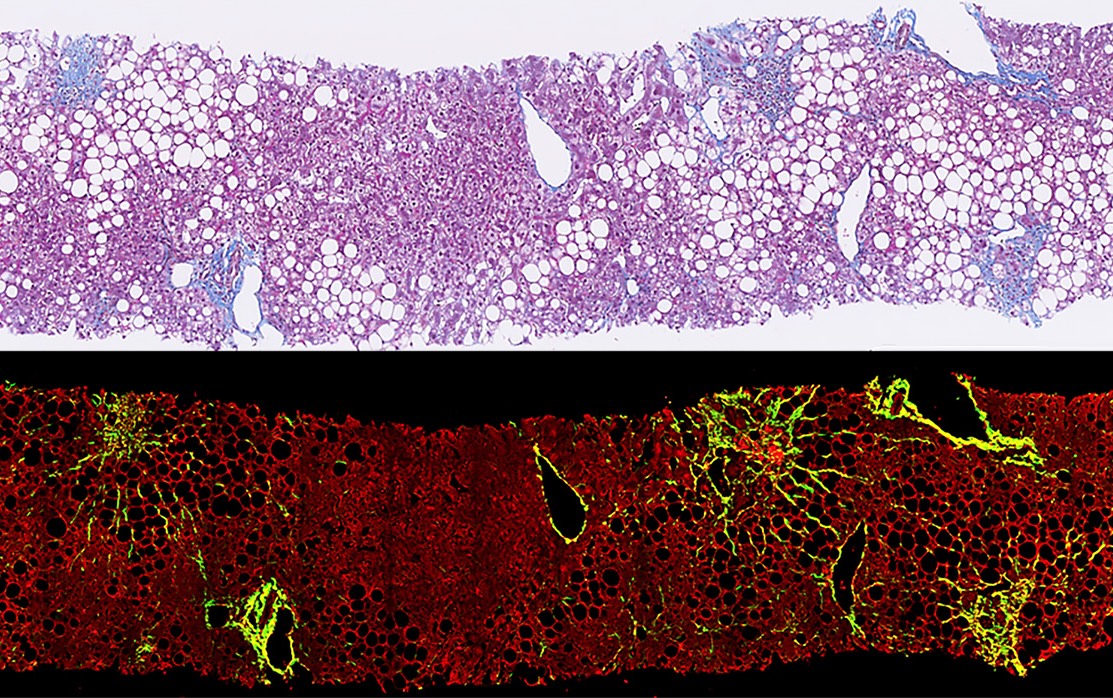
Comparing Stain-Free and Staining Methods
Accurate tissue imaging is essential in studying, diagnosing, and monitoring fibrosis. The choice between conventional staining and stain-free methods often depends on the specific research question, type of tissue, and available resources.
Aspect | Traditional Staining Methods | Stain-Free Imaging Techniques |
---|---|---|
Sample Preparation | Multiple steps: Fixation, dehydration, staining, and mounting | Minimal sample preparation, but may require specialized tissue handling |
Artifacts | Potential staining artifacts, which are well understood and can be controlled | Reduced chemical artifacts but may introduce technique-specific artifacts that are less well-characterized |
Reproducibility | Prone to inter- and intra-observer variability and operator differences | Potentially consistent results, but dependent on equipment calibration |
Visualization | Differentiation of tissue components based on well-understood staining patterns | Visualizes specific structures (e.g., collagen) based on intrinsic optical properties |
Quantification | Ordinal scoring systems (e.g., NASH-CRN, Ishak, METAVIR) widely accepted in clinical practice | Digital image analysis allows for detailed measurements, but clinical validation is still ongoing |
Data Output | Produces familiar images for pathologists; easily interpretable in clinical settings | Produces high-quality, digitized images suitable for computational analysis but may require specialized training |
Clinical Validation | Extensively validated and widely accepted in clinical practice | Promising results in research settings, but clinical validation is still in progress |
- Digital Image Analysis: Stain-free techniques produce high-quality, digitized images that can be readily analyzed using advanced software tools. This digital format facilitates the application of sophisticated image analysis algorithms, which can provide detailed quantitative data on collagen structures and other tissue features2.
- Standardized Assessment: The digital nature of stain-free imaging, combined with automated analysis tools, may help standardize fibrosis assessment across different samples and studies, reducing inter-observer variability and improving the consistency of fibrosis scoring3.
- Preservation of Tissue: Since stain-free methods don’t require chemical staining, the original tissue sample is preserved in its native state. This can be particularly valuable when tissue samples are limited or further analyses (such as molecular studies) are to be performed on the same sample.
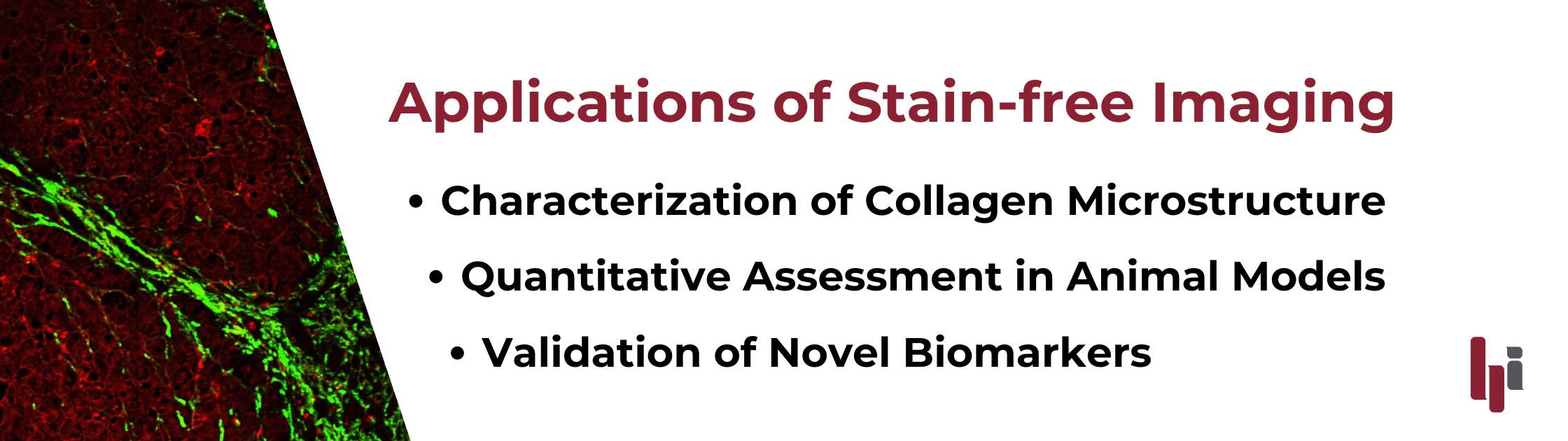
Applications of Stain-Free Imaging
1. Characterization of Collagen Microstructure
- Collagen fiber thickness and density
- Fiber orientation and organization
- Changes in collagen crosslinking
2. Quantitative Assessment of Fibrosis in Animal Models
In preclinical research, stain-free imaging techniques quantitatively assess fibrosis in animal models of various diseases, allowing for:
- More precise measurement of fibrosis progression over time
- Evaluation of anti-fibrotic therapies in development
- Comparison of fibrosis patterns across different animal models
The ability to obtain quantitative data on fibrosis severity and progression can enhance the translational value of animal studies.
3. Validation of Novel Biomarkers.
- Assess the sensitivity and specificity of new biomarkers
- Understand how biomarker levels relate to structural changes in the tissue
- Develop more accurate non-invasive methods for fibrosis assessment
Rezdiffra Case Study
In Madrigal’s Phase IIb MASH trial, qFibrosis revealed that baseline F3 patients were the best responders, with approximately half showing ≥1-point improvement in fibrosis. This data informed Madrigal’s Phase 3 trial design, which included more F3 patients and demonstrated significant improvements in primary endpoints: MASH resolution and fibrosis stage improvement. The AI-based measurements confirmed reductions in fibrosis in Rezdiffra-treated groups, highlighting the efficacy of Madrigal’s treatment.
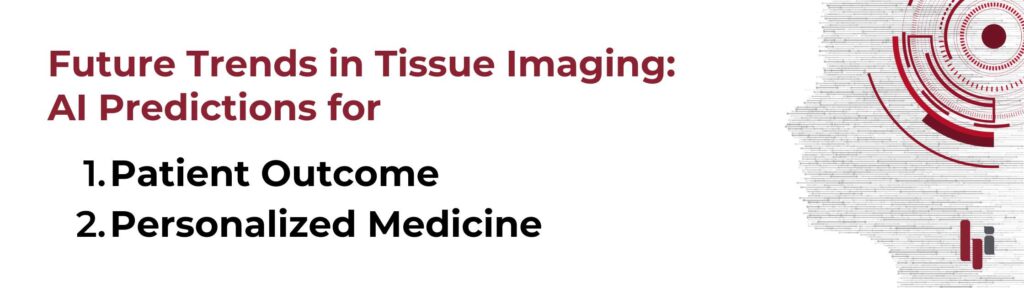
Future Trends in Tissue Imaging
AI-Powered Image Analysis for Patient Outcome Prediction
- Longitudinal Studies: AI can track subtle morphological and architectural changes in tissue structure over time and identify early disease progression indicators that might not be apparent to human observers.
- Multimodal Data Integration: AI algorithms can now integrate stain-free tissue imaging data with patients’ information, such as clinical history, genetic data, and blood biomarkers, for more comprehensive analysis and potentially more accurate predictions5.
- Predictive Modeling: By analyzing patterns in tissue structure alongside patient data, AI models can predict treatment response, disease progression, and long-term patient outcomes, where the rate of progression and response to treatment can vary significantly between patients.
AI-Driven Personalized Medicine in Fibrosis Treatment
- Biomarker Discovery: AI analysis of tissue images may uncover new biomarkers that may unravel information on disease progression, potentially leading to the development of more targeted therapies.
- Treatment Response Prediction: AI algorithms can analyze stain-free images of patient biopsies to identify subtle features or patterns that correlate with positive responses to therapies. This can help trial enrollment and provide the most effective treatment for each patient.
- Designing of Clinical Trials: This AI-driven approach could inform the design of clinical trials, where critical information presented may be considered for trial powering and duration decisions.
FAQs
Stain-free tissue imaging refers to a category of imaging methods that allow for the visualization and analysis of tissue samples without using traditional chemical stains. These techniques, such as Second Harmonic Generation (SHG) microscopy, generate contrast in tissue samples using optical processes.
Unlike conventional methods that rely on chemical stains to highlight tissue structures, stain-free techniques use intrinsic optical properties of tissues to generate contrast. This eliminates the need for staining procedures, which may result in highly variable results due to staining inconsistencies. The tissue is also preserved in its native state for future downstream tests on the same tissue material.
Key applications include characterizing collagen microstructure, quantitative assessment of fibrosis, validating novel biomarkers, and studying fibrosis regression and progression.
While stain-free techniques don't directly quantify fibrosis, they produce high-quality, digitized images with granular details that can be analyzed using advanced software tools. These digital analysis methods can provide detailed quantitative data on finer collagen structures in relation to liver lobule zones.
AI can analyze stain-free tissue images with other patient data to predict outcomes. It can also identify characteristics in patient biopsies that may indicate the likelihood of response to specific treatments, contributing to more personalized approaches in disease management.
Currently, stain-free imaging is seen as complementary to conventional methods rather than a complete replacement. Each approach has its strengths, and researchers often employ multiple techniques to gain comprehensive insights.
Limitations can include the need for specialized equipment, potential difficulties in visualizing certain tissue components that don't generate intrinsic solid signals, and the ongoing need for validation against established methods.
While primarily a research tool at present, stain-free imaging techniques could contribute to more standardized fibrosis assessment, aid in the development of novel biomarkers, and support personalized treatment approaches in the future.
For more information on specific stain-free imaging technologies and their applications in fibrosis research, refer to recent scientific publications or attend relevant conferences. You can also reach out to our team via the Contact page to learn more about our innovative imaging systems.
References
- Sanyal AJ, Jha P, Kleiner DE. Digital pathology for nonalcoholic steatohepatitis assessment. Nat Rev Gastroenterol Hepatol. 2024;21:57-69.
- Ratziu V, Kleiner DE, Bedossa P, et al. Digital pathology and artificial intelligence in non-alcoholic steatohepatitis. J Hepatol. 2023. doi:10.1016/j.jhep.2023.10.015
- Liu F, Goh GB, Tiniakos D, et al. qFIBS: Automated technique for quantitative evaluation in nonalcoholic steatohepatitis. Hepatology. 2020;71(6):1953-1966.
- Ng N, Xiao Y, Leow WQ, et al. Second-harmonic generated quantifiable fibrosis parameters in nonalcoholic fatty liver disease. Clin Pathol. 2023;16:2632010X231162317.
- Kendall TJ, Duff CM, Thomson SJ, et al. Integrated gene-to-outcome database for metabolic dysfunction-associated steatotic liver disease. Nat Commun. 2023;14(1):3918.