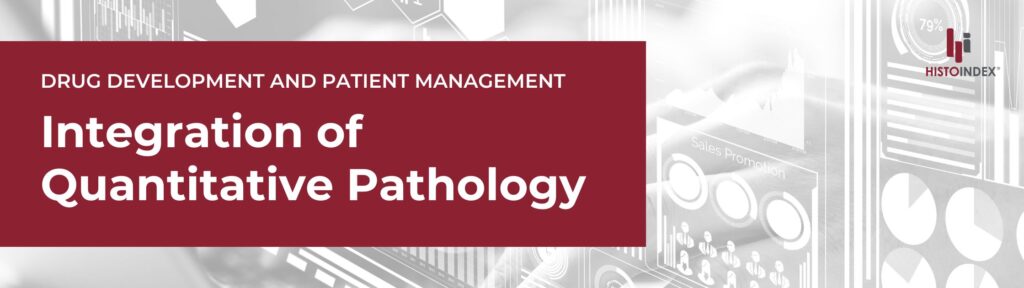
Introduction
Pathology, the cornerstone of medical diagnosis, has witnessed a transformative evolution from its traditional roots to leveraging cutting-edge digital technology. This shift has not only refined diagnostic accuracy but has fundamentally altered the landscape of medical research, clinical trials, and patient care.
Quantitative pathology, a pivotal component of this digital transition, employs advanced imaging and analysis techniques to measure and interpret biological information in ways that were once beyond reach. Unlike traditional pathology, which often relies on subjective interpretation, quantitative pathology offers a more precise, data-driven approach. This methodology enhances our understanding of disease mechanisms, potentially leading to more effective treatments and personalized patient care strategies.
Despite advancements and the growing reliance on quantitative pathology in medical settings, significant gaps remain. The need for continuous development is evident as the medical community strives to address challenges such as data integration, standardization of analysis protocols, and the training required to maximize the potential of digital tools.
As we investigate quantitative pathology’s capabilities and impacts, we will explore not only its current applications but also future opportunities for enhancing drug development and improving patient treatment outcomes.
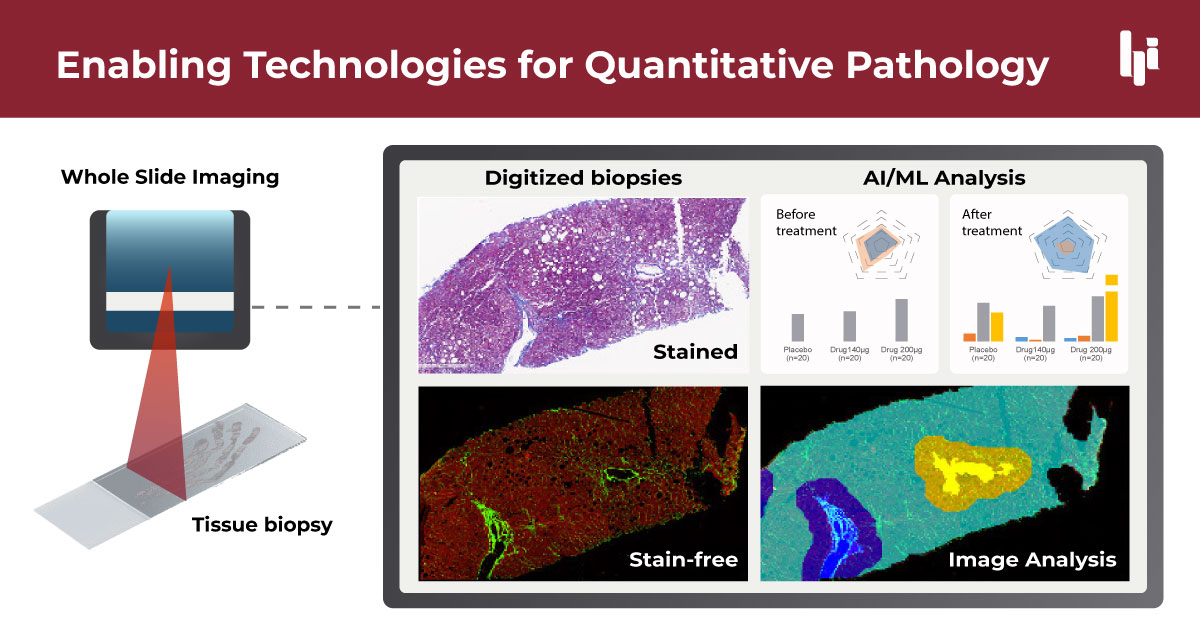
Enabling Technologies for Quantitative Pathology
Quantitative pathology relies on several key enabling technologies that have transformed traditional pathology practices. Digital pathology and whole slide imaging (WSI) form the foundation of this approach. WSI systems capture high-resolution digital images of entire tissue slides, allowing for detailed examination and analysis without the physical slides. These digital images can be easily shared, stored, and accessed remotely, facilitating collaboration among pathologists and researchers.
Image analysis software represents the next layer of technology, employing various techniques to detect, measure, and quantify tissue features and biomarkers. These methods include thresholding, which separates objects from the background based on pixel intensity; segmentation, which divides images into multiple segments or objects; and morphological operations, which analyze and process geometric structures in the image. These tools excel at extracting precise, reproducible measurements from images based on predefined parameters and rules. Image analysis is valuable for standardized tasks such as characterizing collagen fibers, counting inflammatory cells, and evaluating ballooning from degeneration of hepatocytes in large datasets of biopsy images quickly and consistently.
Artificial intelligence (AI) and machine learning (ML), particularly deep learning models, represent a more advanced form of analysis. Unlike traditional image analysis, which relies on predefined rules, AI systems can learn to recognize complex patterns and make predictions based on vast amounts of training data. These AI-driven approaches can perform more sophisticated tasks such as automatically classifying tissue types, detecting subtle patterns associated with disease progression, or even predicting patient outcomes. AI has the potential to uncover novel insights that might not be apparent through traditional analysis methods.
While traditional image analysis excels at specific, rule-based tasks, AI can discover novel relationships in data and handle more variable or ambiguous scenarios. AI systems complement traditional image analysis by leveraging large datasets to identify complex patterns and make predictions. This helps clinicians obtain more objective, quantifiable information from tissue samples, supporting more precise diagnoses, and better-informed treatment decisions, and also helps drug development companies design more rigorous clinical trials.
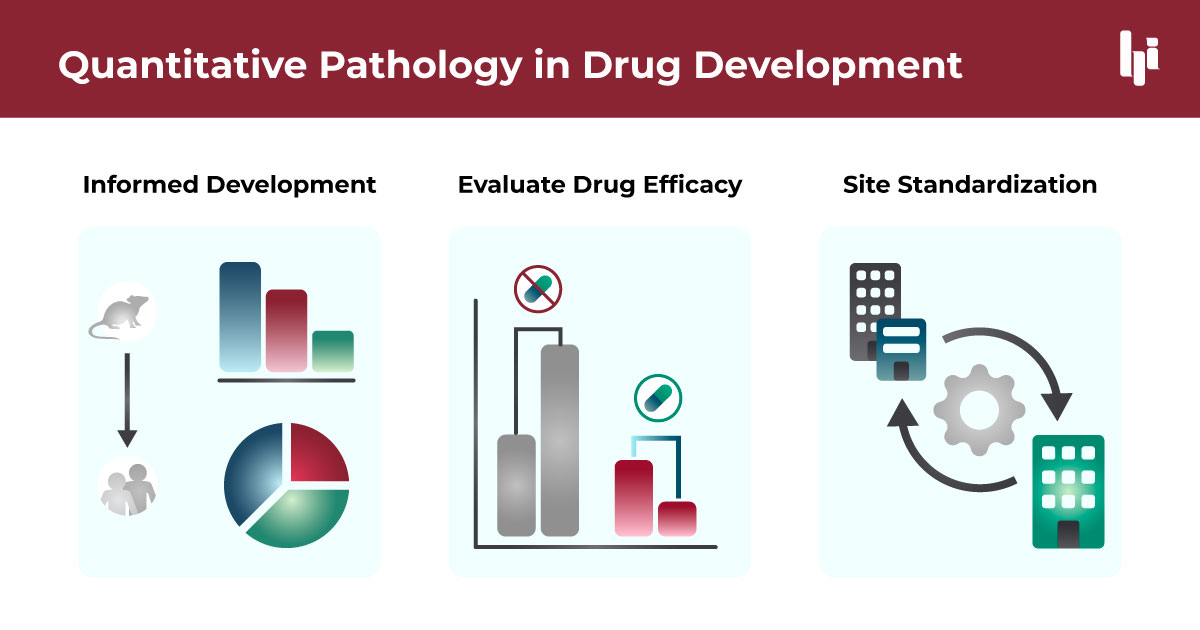
Applications in Clinical Trials Drug Development
Quantitative pathology plays a pivotal role in clinical trials and drug development, particularly through its ability to precisely track disease progression and regression. This capability is crucial for assessing the efficacy of new drugs, providing a robust framework to objectively measure therapeutic impacts in clinical settings.
The integration of digital pathology with artificial intelligence (AI) analyses has opened new avenues for deeper insights into specific treatment effects, such as fibrosis regression in diseases like nonalcoholic steatohepatitis (NASH). Digital pathology combined with AI can effectively quantify fibrosis regression, a key indicator of therapeutic success in NASH treatments, to provide greater granularity into treatment-induced changes4.
Quantitative pathology also enhances the drug development process across various clinical trial phases. In early-phase trials (Phase I and II), it enables precise assessment of drug effects on target tissues, helping researchers identify promising drug candidates and optimal dosing regimens. This precision can lead to more informed go/no-go decisions, potentially saving time and resources by early termination of less promising compounds. As trials progress to Phase III, quantitative pathology provides robust, reproducible endpoints that can strengthen regulatory submissions. It allows for a more nuanced evaluation of drug efficacy, potentially uncovering subpopulations that show enhanced response to treatment. This capability is particularly valuable in the era of precision medicine, where it can guide the development of companion diagnostics alongside new therapeutics, ultimately improving the likelihood of successful drug approval and targeted patient treatment strategies.
Another key advantage of quantitative pathology in drug development is its ability to facilitate the design and optimization of clinical trials across different study sites. By ensuring standardization and consistency in how samples are analyzed across multiple study sites, quantitative pathology minimizes variability that can skew results, thereby enhancing the reliability of trial outcomes. This standardization is especially important when trials are scaled up or when they involve multiple international sites, ensuring that data comparability is maintained regardless of the location.
These technological advancements not only improve the accuracy of clinical trial assessments but also accelerate the development process by enabling quicker, more reliable decisions about the progression between trial phases. As such, quantitative pathology is becoming an indispensable tool in the drug development arsenal, promising to enhance the speed and precision of bringing new therapeutic options to the market.
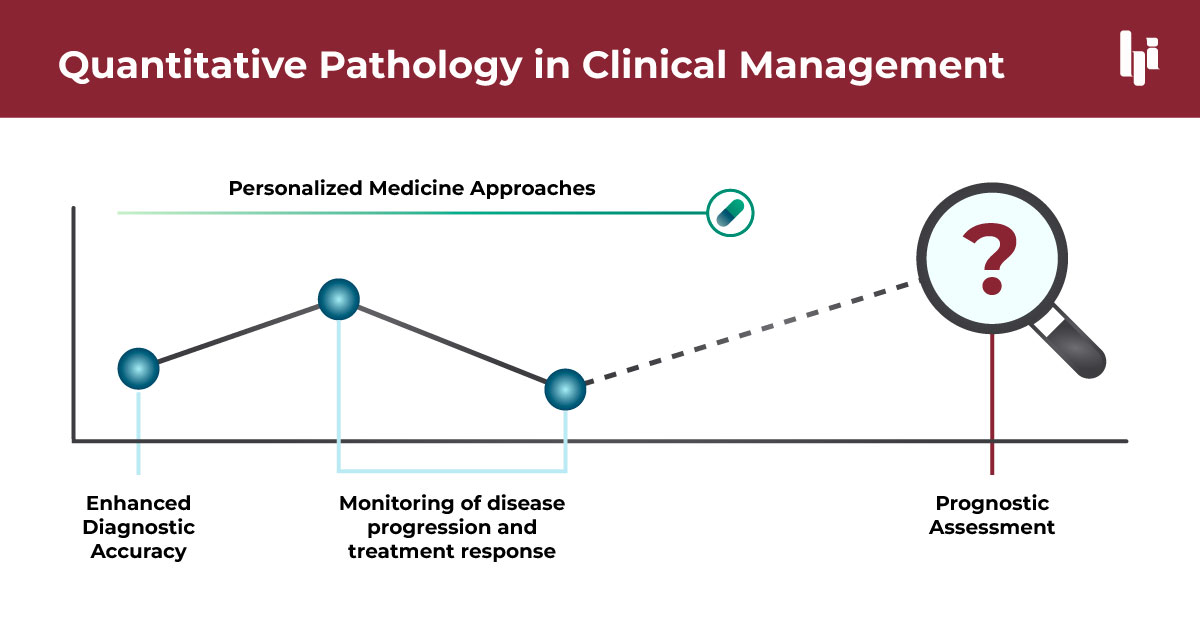
Applications in Clinical Patient Management
Quantitative pathology significantly enhances clinical diagnosis and patient management by providing improved diagnostic accuracy and consistency. This technological advancement allows for a more detailed and objective evaluation of pathological samples, facilitating enhanced diagnostic precision.
Enhanced Diagnostic Accuracy and Consistency: The integration of quantitative methods, such as qFibrosis, enables a more standardized assessment of tissue samples. The precision of these tools aids in reducing variability among interpretations by different clinicians, ensuring consistency in diagnosis across various settings.
Prognostic Assessment: Quantitative pathology, especially techniques like qFibrosis, has shown great promise in prognostic assessments. Research has demonstrated its effectiveness in predicting outcomes such as death and decompensated cirrhosis, making it a valuable tool for guiding treatment decisions. The ability to accurately predict disease progression supports personalized treatment planning, enhancing the likelihood of successful outcomes.
Supporting Personalized Medicine Approaches: The detailed data obtained from quantitative pathology facilitate personalized medicine strategies. By understanding the unique morphological characteristics of an individual’s fibrosis architecture, treatments can be tailored specifically to their profile, improving therapeutic efficacy and patient outcomes.
Monitoring Disease Progression and Treatment Response: These advanced methodologies are crucial for monitoring disease progression and evaluating patient response to treatment. By providing quantitative data on fibrosis changes over time, clinicians can make informed decisions about the effectiveness of current treatment protocols and make necessary adjustments.
This integration of advanced quantitative pathology technologies not only supports existing clinical practices but also paves the way for innovative approaches in personalized medicine, significantly altering the landscape of patient management and treatment planning.
HistoIndex's Role in Advancing Drug Development
HistoIndex’s innovative technologies, particularly its stain-free imaging and AI-driven qFibrosis analysis, have revolutionized drug development in clinical trials. Their technology was integral in the Phase 2 and Phase 3 trials of Rezdiffra™ for treating MASH, where it served as an exploratory endpoint. The precise quantification of fibrosis by HistoIndex allowed for more accurate assessments of drug efficacy. Furthermore, their AI-enhanced digital pathology tools have improved pathologist concordance in fibrosis staging, enhancing the reliability and speed of clinical evaluations. These contributions have proven pivotal in advancing therapeutic developments and achieving regulatory milestones.
Challenges in Adoption and Future Perspectives
Adopting new quantitative pathology technologies presents several challenges for drug companies and research groups. Key among these is the integration of AI, which involves overcoming hurdles such as high initial costs, complexity of implementation, and a shortage of skilled personnel to manage and interpret AI outputs. Additionally, the current limitations of quantitative pathology techniques include variability in data interpretation, the need for standardized protocols, and technological integration with existing systems.
Efforts to address these challenges include ongoing technological advancements, increased training programs for pathologists, and the development of more robust AI algorithms that can handle diverse data sets and provide more consistent results. Looking forward, the future of quantitative pathology promises significant advancements in drug development and clinical trials. Enhanced precision in drug efficacy testing, improved patient stratification, and faster regulatory approvals are anticipated as these technologies mature and become more integrated into mainstream research and healthcare settings.
Partnering with Histoindex
Research groups and pharmaceutical companies dedicated to combating fibrotic diseases are invited to consider HistoIndex as their partner. With a proven track record in enhancing the precision of clinical trials through advanced, AI-enhanced quantitative pathology technologies, HistoIndex is poised to expand our impact beyond NASH to a variety of fibrotic conditions. Our accurate, efficient, and stain-free solutions are designed to support complex studies, paving the way for groundbreaking advancements in medical research. Connect with us today to get started or learn more.
FAQs
Quantitative pathology involves the use of advanced imaging and computational technologies to analyze tissue samples. This approach allows for precise measurement and analysis of pathological features, enhancing the accuracy and consistency of diagnoses and research findings.
Quantitative pathology enhances drug development by providing accurate, reproducible data that can track disease progression and regression. This data is crucial for assessing the efficacy of treatments in clinical trials, allowing for better-informed decisions on drug safety and effectiveness.
Integrating AI with digital pathology allows for more sophisticated analysis of medical images. AI algorithms can detect patterns and provide insights that are not easily visible to human observers, leading to a deeper understanding of disease mechanisms and treatment effects.
Challenges include the high cost of advanced imaging equipment, the need for specialized training for personnel, integration with existing systems, and handling large volumes of data produced by these technologies.
Yes, while the focus is often on fibrotic diseases like NASH, quantitative pathology has applications across a range of diseases where tissue analysis is crucial, including cancers, inflammatory disorders, and other chronic conditions.
The future of quantitative pathology looks promising, with ongoing advancements in AI and imaging technologies expected to further refine its capabilities. This will likely lead to more personalized medicine approaches, where treatments are tailored based on detailed pathological data.
Quantitative pathology provides detailed data that can help identify the specific characteristics of a patient’s disease, enabling healthcare providers to tailor treatments that are more likely to be effective for individual patients, thus supporting the broader goals of personalized medicine.
References
- Hsiao, Chih-Yang, et al. “Potential of using qFibrosis analysis to predict recurrent and survival outcome of patients with hepatocellular carcinoma after hepatic resection.” Oncology (2024).
- Abdurrachim, Desiree, et al. “Validation of the HI AI-DP Platform as an Aiding Tool to Increase Pathologist Concordance on Fibrosis Staging in NASH.” AASLD The Liver Meeting (2023)
- Naoumov, Nikolai V., et al. “Digital pathology with artificial intelligence analyses provides greater insights into treatment-induced fibrosis regression in NASH.” Journal of Hepatology 77.5 (2022): 1399-1409.
- Sanyal, Arun J., Prakash Jha, and David E. Kleiner. “Digital pathology for nonalcoholic steatohepatitis assessment.” Nature Reviews Gastroenterology & Hepatology 21.1 (2024): 57-69.